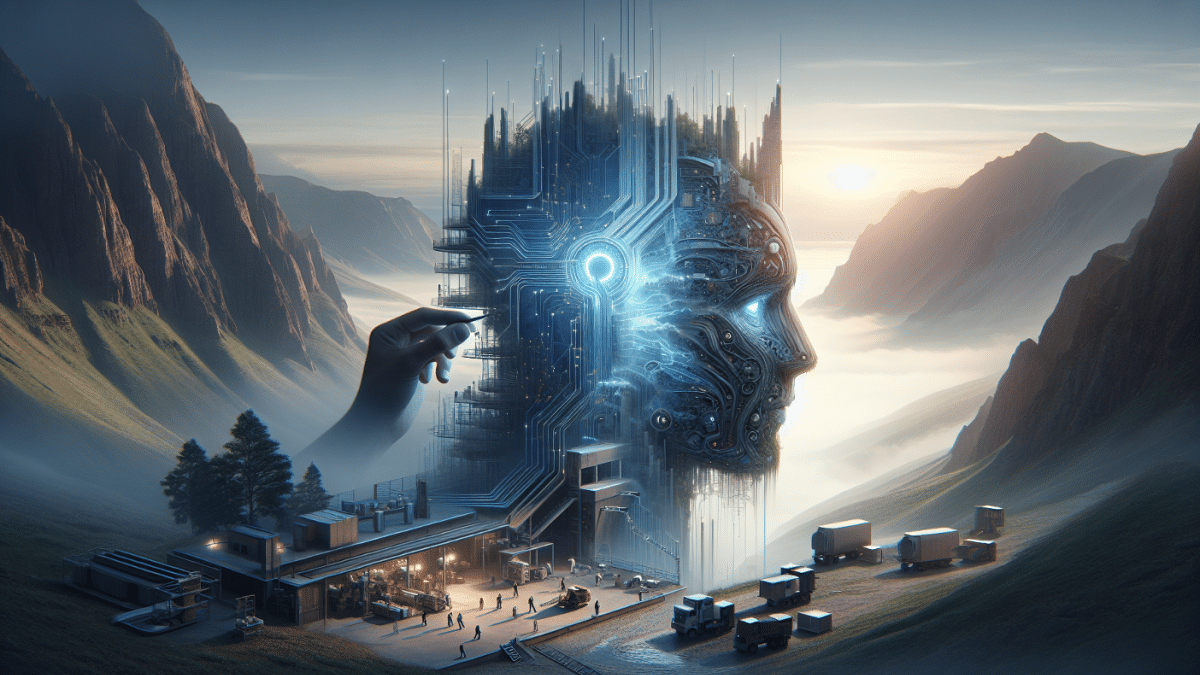
Overview of DeepSeek R1
DeepSeek R1 is an advanced AI model designed for data analysis and pattern recognition. Using deep learning techniques, it processes complex datasets to deliver actionable insights and accurate predictions. This model is widely applied in industries such as healthcare, finance, and technology to improve decision-making and operational workflows.
Data Requirements for Effective Implementation
1. Data Quality
High-quality data is essential for training DeepSeek R1. The datasets must be comprehensive, accurate, and well-labeled to ensure effective learning and reliable results.
2. Preprocessing
Proper data preprocessing enhances the relevance and usability of the data. This step involves cleaning, normalizing, and structuring the data to meet the model’s requirements.
Hyperparameter Tuning
1. Optimizing Model Performance
Adjusting hyperparameters is a critical step in improving the performance of DeepSeek R1. This process fine-tunes the model to meet specific application needs and ensures it operates efficiently.
2. Implementation Strategies
Hyperparameter tuning involves experimenting with different configurations to identify the optimal settings for the model. This step is key to achieving high accuracy and performance in real-world scenarios.
Evaluating Model Performance
1. Performance Metrics
Evaluating the model involves using specific metrics to measure its accuracy, precision, recall, and overall effectiveness. These metrics ensure the model meets the required standards for deployment.
2. Iterative Fine-Tuning
Continuous evaluation and fine-tuning are necessary to optimize the model. This process adjusts parameters based on performance results, improving output quality and reliability.
Deployment and Integration
1. System Integration
After training and fine-tuning, DeepSeek R1 is integrated into existing systems to begin delivering value. Proper integration ensures the model operates seamlessly within the workflow.
2. Deployment Best Practices
Effective deployment involves testing the model in real-world conditions to confirm its reliability and accuracy. This step ensures that the model performs as expected once fully operational.
Monitoring and Maintenance
1. Continuous Monitoring
Post-deployment monitoring tracks the model’s performance over time. This step identifies any drifts, performance drops, or issues that may arise during real-world use.
2. Ongoing Maintenance
Regular updates and retraining with new data ensure the model adapts to evolving requirements. Maintenance keeps DeepSeek R1 relevant and effective as conditions change.
Planning for Future Scaling
1. Adapting to Larger Datasets
As datasets grow, DeepSeek R1 must be scaled to handle increased data volumes. Scaling can be achieved through horizontal scaling (adding more systems) or vertical scaling (upgrading existing hardware).
2. Staying Updated
Regular updates ensure the model benefits from the latest advancements in AI. Monitoring new developments and incorporating updates keeps DeepSeek R1 aligned with industry standards and technological progress.
Long-Term Value of DeepSeek R1
DeepSeek R1’s capabilities in data analysis, pattern recognition, and decision-making make it a valuable tool for businesses. By focusing on data quality, performance optimization, and continuous improvement, this model delivers reliable results across various applications and industries.